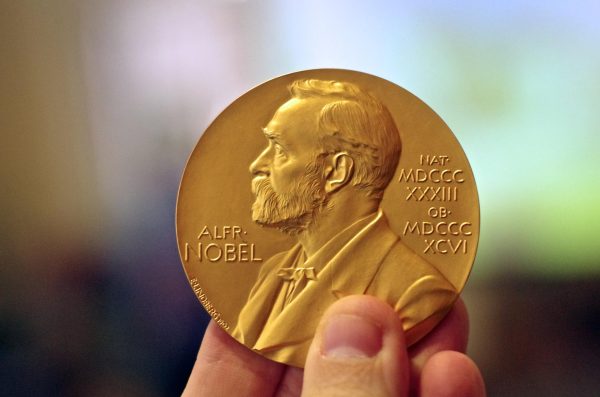
This year’s Nobel Prize in Chemistry went to David Baker from the University of Washington for computational protein design and John Jumper and Demis Hassabis from Google Deepmind for protein structure prediction. They discovered the code to complex protein structure and used it to build new proteins. The Nobel Prize highlights the significant role Artificial Intelligence (AI) has played in advancing profound discoveries in Chemistry. The Nobel Prize reminds us that we are standing at the gates of a new era in scientific discovery – led by AI, that promises to accelerate our understanding of biochemistry, disease mechanisms, and advance drug discovery. While there is much to celebrate, AI in science introduces new ethical challenges for modern scientists.
Jumper and Hassabis discovered AI methods to predict the structure of proteins in the human body, using AlphaFold. AlphaFold is an AI model built using a mathematical system called a neural network; this network searches and analyzes large amounts of protein data to identify sequences iteratively. AlphaFold is able to search known databases of existing simpler amino acids (proteins) and use this information to predict the structure of long and complex protein molecules. The Neural Network then creates hypothetical sequences of complex proteins and discards ones that are improbable. This method is used to create previously unknown protein structures.
Knowledge about protein structure is critical to understand diseases. Alteration of protein structure is at the heart of cancer, several infectious and degenerative diseases. Repairing and restoring the structure leads to better drugs that are more effective and cause less side effects. Additionally, AlphaFold has predicted the structure of all the proteins in the human body, significantly adding to the amount of data available regarding protein structure. This data can now be mined to create drugs that have the potential to break up harmful proteins like amyloid fibrils in Alzheimers.
AI has played a significant role in advancing scientific discovery. Predicting the structure of a protein would have taken many laborious years of scientific experimentation. For example, it took 22 years of work to predict the structure of myoglobin, a protein found in muscles. With AlphaFold, this process is sped up dramatically through the use of computer simulations by creating a virtual model that can predict protein structures without actually having to synthesize that complex protein molecule in the lab. Scientists can now analyze large amounts of data, extract hidden patterns unseen and not discoverable to the human eye, and quickly create alternate realities with speed and accuracy. This reduces effort pursuing failed experiments.
Alpha Fold would not have been created without computer engineering (neural networks), computational biology (protein sequencing), and bioinformatics (creating new protein structures). Additionally, it will go on to assist researchers, pharmaceutical scientists, and chemical engineers. This collaborative approach will continue to assist in major findings in the future.
Although AI has the ability to uncover much good, the potential for misuse and harm still exists. The use of AI raises questions about accountability. Who is to blame when the technology goes wrong? There is also the question of whether the public can count on industry to be transparent about AI failures.
Data fed into AI modules can be easily, unknowingly or deliberately biased, thus creating disadvantages for certain populations. The scientific society should develop ethical processes and code of conduct to avoid unfortunate complications. Such processes should emphasize clear protocols for data collection, AI training, and privacy.
Beyond drug discovery, AI is assisting major breakthroughs in genomics, imaging, autonomous driving, space exploration, climate modeling, and nanotechnology. The potential of AI is exhilarating, and the next decade of scientists can use this technology for astounding revelations, both in science and the world at large.